Matrix Solver Reduced Row Echelon Form
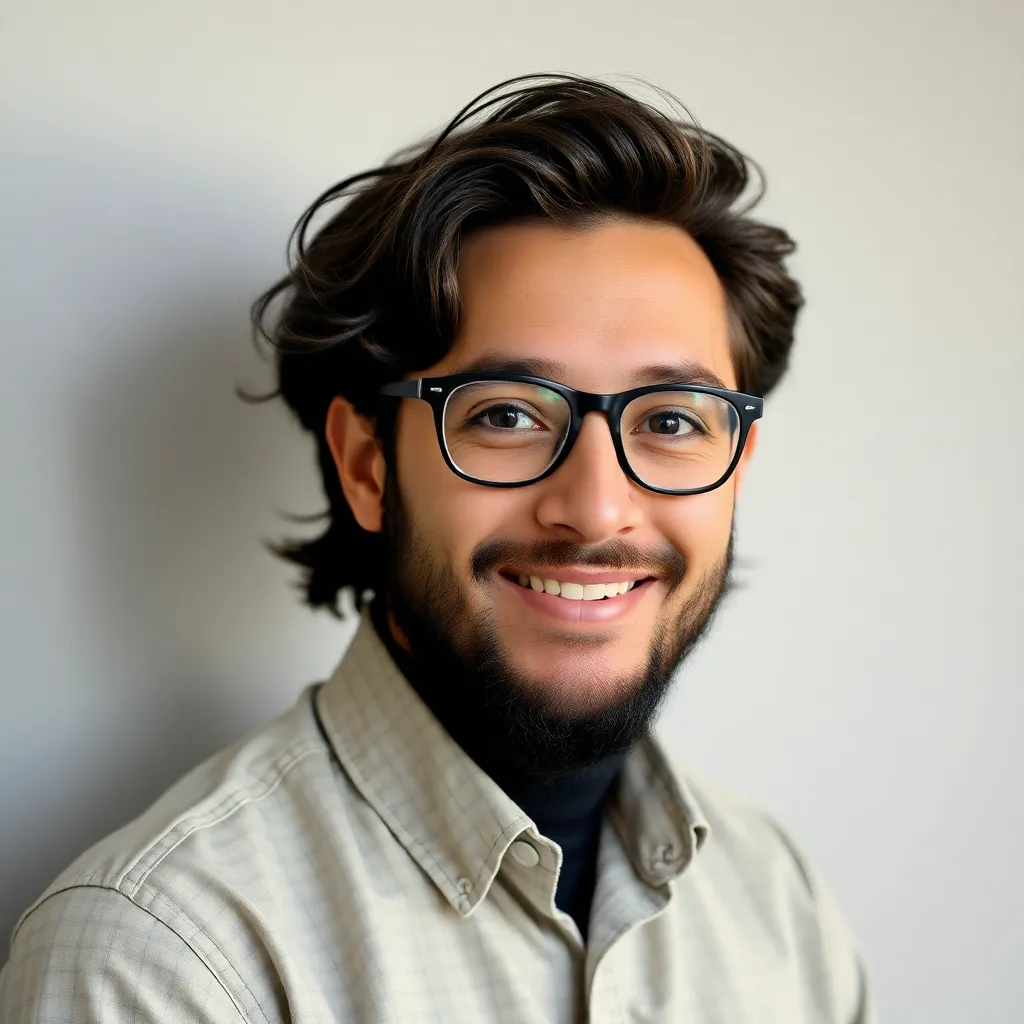
Greels
Apr 08, 2025 · 6 min read

Table of Contents
Matrix Solver: A Deep Dive into Reduced Row Echelon Form
Solving systems of linear equations is a fundamental task in various fields, from computer graphics and engineering to economics and machine learning. Matrices provide a powerful and efficient way to represent and solve these systems. One of the most effective methods for solving systems of linear equations represented by matrices is through the process of finding the reduced row echelon form (RREF). This article provides a comprehensive guide to understanding and applying RREF for solving matrices.
Understanding Matrices and Systems of Linear Equations
A matrix is a rectangular array of numbers, symbols, or expressions, arranged in rows and columns. A system of linear equations can be concisely represented using a matrix, where each row represents an equation, and each column represents a variable. For example:
2x + y - z = 8 -3x - y + 2z = -11 -2x + y + 2z = -3
This system can be represented by the augmented matrix:
[ 2 1 -1 | 8 ]
[-3 -1 2 |-11]
[-2 1 2 |-3 ]
The goal is to find the values of x, y, and z that satisfy all three equations simultaneously.
What is Reduced Row Echelon Form (RREF)?
The reduced row echelon form is a specific format for a matrix that simplifies the solution process. A matrix is in RREF if it satisfies the following conditions:
- Leading entries (the first non-zero element in each row) are 1.
- Each leading 1 is the only non-zero entry in its column.
- Rows with all zeros are at the bottom of the matrix.
- The leading 1 in each non-zero row is to the right of the leading 1 in the row above it.
Transforming a matrix into its RREF essentially solves the corresponding system of linear equations. The solution is directly readable from the RREF matrix.
Elementary Row Operations: The Tools for Reaching RREF
The transformation of a matrix into RREF is accomplished using elementary row operations. These are fundamental manipulations that don't alter the solution set of the corresponding system of equations. These operations include:
- Swapping two rows: Interchanging the position of any two rows.
- Multiplying a row by a non-zero scalar: Multiplying all entries in a row by the same non-zero constant.
- Adding a multiple of one row to another row: Adding a scalar multiple of one row to another row.
By strategically applying these operations, we systematically transform the matrix into RREF.
Step-by-Step Guide to Finding RREF
Let's illustrate the process with the example matrix:
[ 2 1 -1 | 8 ]
[-3 -1 2 |-11]
[-2 1 2 |-3 ]
Step 1: Create a leading 1 in the first row.
Divide the first row by 2:
[ 1 0.5 -0.5 | 4 ]
[-3 -1 2 |-11]
[-2 1 2 |-3 ]
Step 2: Create zeros below the leading 1 in the first column.
Add 3 times the first row to the second row, and add 2 times the first row to the third row:
[ 1 0.5 -0.5 | 4 ]
[ 0 0.5 0.5 | 1 ]
[ 0 2 1 | 5 ]
Step 3: Create a leading 1 in the second row.
Multiply the second row by 2:
[ 1 0.5 -0.5 | 4 ]
[ 0 1 1 | 2 ]
[ 0 2 1 | 5 ]
Step 4: Create zeros above and below the leading 1 in the second column.
Subtract 0.5 times the second row from the first row, and subtract 2 times the second row from the third row:
[ 1 0 -1 | 3 ]
[ 0 1 1 | 2 ]
[ 0 0 -1 | 1 ]
Step 5: Create a leading 1 in the third row.
Multiply the third row by -1:
[ 1 0 -1 | 3 ]
[ 0 1 1 | 2 ]
[ 0 0 1 |-1 ]
Step 6: Create zeros above the leading 1 in the third column.
Add the third row to the first row, and subtract the third row from the second row:
[ 1 0 0 | 2 ]
[ 0 1 0 | 3 ]
[ 0 0 1 |-1 ]
The matrix is now in RREF.
Interpreting the RREF Solution
The RREF matrix directly provides the solution to the system of linear equations. Each row represents an equation. In this case:
x = 2 y = 3 z = -1
This is the unique solution to the original system of equations.
Cases with Infinite Solutions and No Solutions
Not all systems of linear equations have a unique solution. When solving using RREF, we can encounter two other possibilities:
-
Infinite Solutions: If the RREF matrix contains a row of zeros, except for the last entry (which is also zero), then the system has infinitely many solutions. This indicates dependent equations.
-
No Solutions: If the RREF matrix contains a row of zeros except for the last entry (which is non-zero), then the system has no solutions. This indicates inconsistent equations.
Applications of RREF and Matrix Solvers
The reduced row echelon form and the process of Gaussian elimination (the algorithm used to find RREF) have extensive applications across numerous fields:
- Computer Graphics: Transforming points and objects in 3D space.
- Engineering: Solving systems of equations arising in structural analysis, circuit analysis, and fluid mechanics.
- Economics: Analyzing input-output models and solving economic equilibrium problems.
- Machine Learning: Solving least squares problems in regression analysis.
- Cryptography: Solving systems of linear congruences in cryptography.
- Network Analysis: Analyzing flow in networks.
Advanced Topics and Considerations
While the basic principles of RREF are straightforward, several advanced aspects deserve mention:
- Computational Efficiency: For large matrices, the computational cost of finding RREF can be significant. Optimized algorithms and specialized software are often employed to handle such cases efficiently.
- Numerical Stability: Rounding errors can accumulate during the calculations, leading to inaccuracies in the solution. Sophisticated techniques are employed to mitigate these errors.
- Software and Tools: Numerous software packages (Matlab, Python with NumPy/SciPy, etc.) provide efficient functions for calculating RREF.
Conclusion
The reduced row echelon form is a powerful tool for solving systems of linear equations. Understanding the principles behind RREF, the elementary row operations, and the interpretation of different solution cases (unique solution, infinite solutions, no solution) is crucial for anyone working with matrices and linear algebra. Its applications are wide-ranging, making it an essential concept in various scientific and engineering disciplines. By mastering RREF, you unlock a powerful technique for solving complex mathematical problems and analyzing data effectively. The efficiency and clarity it offers make it an indispensable tool in the world of linear algebra and beyond. The ability to interpret the results, understanding the nuances of unique solutions versus infinite solutions or no solutions, is crucial for applying this technique effectively in real-world scenarios. With practice and a solid understanding of the underlying principles, the power of RREF can be harnessed to tackle a wide array of challenging problems.
Latest Posts
Latest Posts
-
7 Kilograms Equals How Many Pounds
Apr 16, 2025
-
What Is 120 In In Feet
Apr 16, 2025
-
How Many Feet Are In 40 In
Apr 16, 2025
-
What Is 32 Ounces In Pounds
Apr 16, 2025
-
What Is 3 9 In Inches
Apr 16, 2025
Related Post
Thank you for visiting our website which covers about Matrix Solver Reduced Row Echelon Form . We hope the information provided has been useful to you. Feel free to contact us if you have any questions or need further assistance. See you next time and don't miss to bookmark.